Artificial Intelligence (AI) and Machine Learning (ML) can return significant value to factories, but there are still challenges to overcome. These challenges are not in the data science – the code usually works well. The primary challenges to delivering AI/ML projects at scale are rooted in architecture and data governance. Manufacturers need to find an efficient, and cost-effective way to find, understand, use & then reuse data across multiple projects.
Leading manufacturers have begun to embrace the four principles of a data mesh, even if it is not referred to as a data mesh. Their aim is to evolve their architecture into one that supports delivering Industry 4.0 projects at scale and avoiding the two most common modes of failure.
Initial failures were labelled ‘pilot purgatory’. Here the primary mode of failure was technology scaling: what worked in the lab did not always scale to support the demands of the production environment.
Once the technology scaling issue was rectified, ‘use case purgatory’ emerged as a new failure mode. Here the challenge is resource scaling. Companies were delivering Industry 4.0 solutions using an Industry 3.0 architecture. In other words, creating a dedicated pipeline to feed an application which supports a single, or few use cases. In this purgatory, use cases are deployed, but at a high price. The development costs of a dedicated data pipeline coupled with support cost of maintaining isolated apps means this purgatory is not filled with failure, it is sparsely populated. Additional use cases are out of reach due to limited budget.
The clear path out of use case purgatory is a better use of resources – and in this case the key resources are data and data science skills. In a factory environment the amount and variety of data is large and growing. However, it is also finite – and crucially multipurpose. For example, sensor data that comes from a machine can support maintenance use cases, provide insight into the quality of products produced and support process improvement. Machine data is both a large data set and needs very specific operator knowledge to provide context to measurements, alerts, and error codes. To gather and preparing this set of data multiple times is a waste of data science resources. Why not collect, cleanse and pre-process it once for all three use cases, plus others?
This is why the 4 principles of a data mesh should help make better use of limited resources. The first two principles describe a multipurpose approach to data cleansing.
Firstly, domain-driven ownership of data will ensure those people who really understand contextual data are given responsibility to prepare this for multiple use cases. As OT and IT have traditionally been separate in a manufacturing company, the ownership principle should be relatively easy to implement.
Secondly, data must be treated as a product. Creating data products is a new approach, but one that should be readily embraced given the use case purgatory experience. Data products can be as simple as data sets prepared for analytic purposes, or more complex such as the output of ML routines.
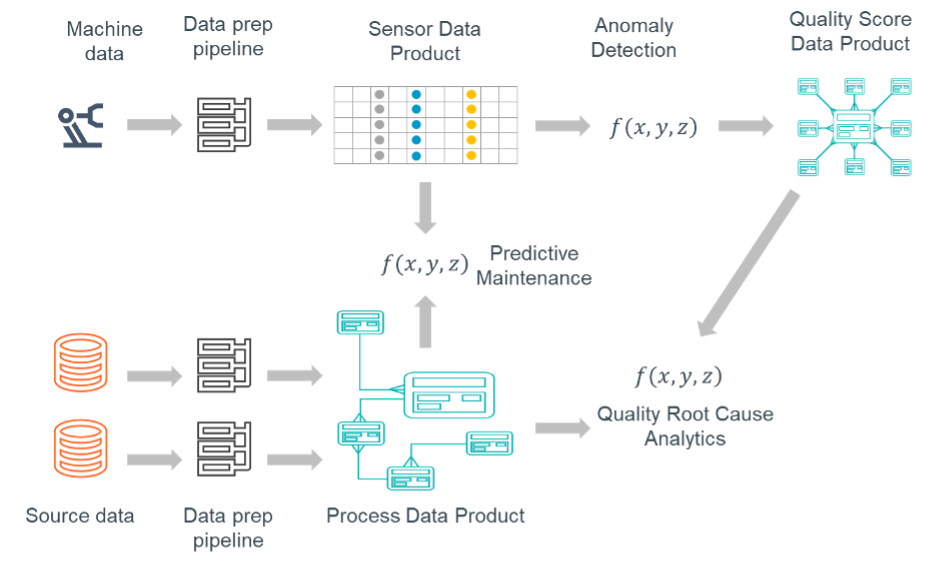
These two principles do not require specific technology, the main implication is organizational. Distributed groups must accept responsibility for ownership of a specific set of data – and ensuring this is available to all authorized users. This has added benefits in terms of reducing cost and increasing security by reducing data movements and redundancies.
Thirdly, a self-serve data infrastructure as a platform needs to be implemented. This is crucial to allowing a variety of teams to build on the work of others to create their own insights from data, as well as additional data products.
And fourthly, the federated computational governance requires a blend of mesh-oriented governance practice and the ability to automate some tasks such as schema or lineage creation.
The final two principles do imply some technical capabilities or tools. At Teradata, we have ongoing investment in technical capabilities to find, understand, use and re-use data – regardless of where it is stored. This is perfectly aligned with implementing a data mesh. Instead of having to move data at a high cost, we can implement an open data ecosystem and bring analytics to the data.
This open ecosystem will also help manufacturers achieve the ideal of good, quick, and cheap in their Industry 4.0 analytics projects:
Good: Increased use case deployment based on trusted & secure data
Quick: Speed of use case deployment & use case execution
Cheap: Limit data movements and redundancies, improve compute efficiency
Manufacturing operations, supported by both IT and OT are not too far away from implementing the data mesh principles. To reap the full potential of AI and ML within a factory setting only small adjustments are required: Data governance practice to adapt ownership and introduce data products, coupled with highly scalable compute power able to operate efficiently in a diverse data ecosystem are the next steps.